From 27th to 30th of June 2017 the 12th USA/EUROPE Air Traffic Management Research & Development Seminar took place in Seattle, Washington, USA. 148 registered participants from 19 countries were counted.
It was a collaborative event of the Federal Aviation Administration (FAA), Eurocontrol and the American Institute of Aeronautics and Astronautics (AIAA). The MALORCA project team led by Prof. Hartmut Helmke (DLR) and assisted by Oliver Ohneiser (DLR) and Christian Kern (ACG) in cooperation with Jörg Buxbaum (DFS) provided a paper to be presented in the Human Factors track of the seminar. We are proud to report that our paper titeled “Increasing ATM Efficiency with Assistant Based Speech Recognition” was awarded best paper in the Human Factors track.
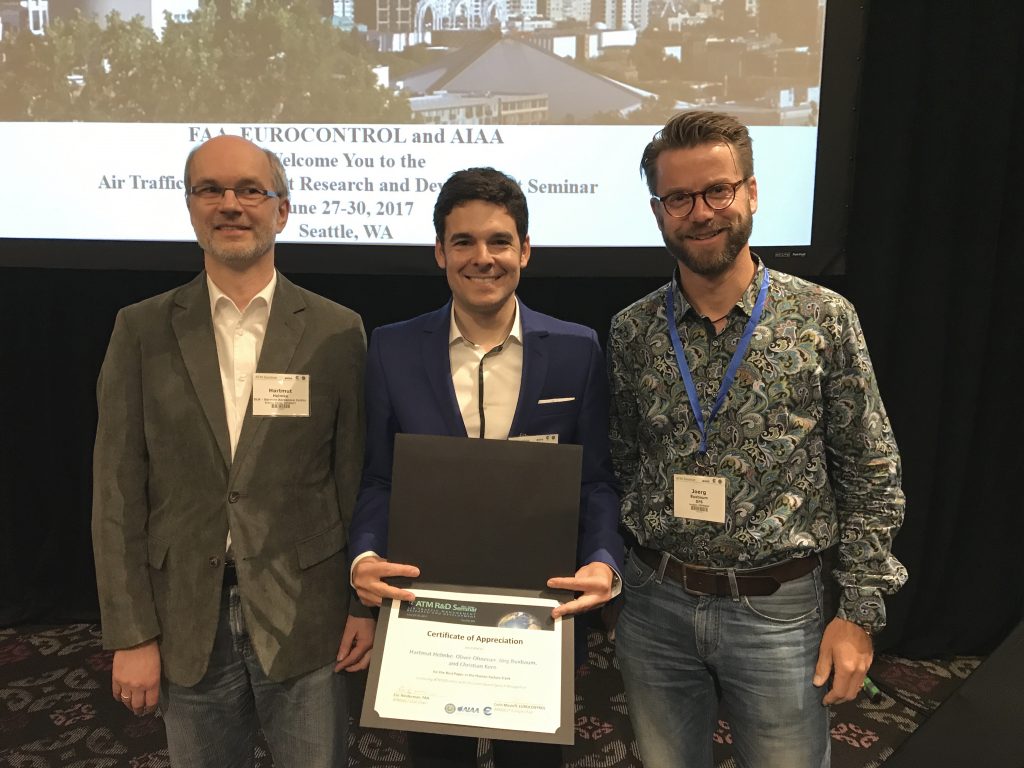
We feel very honored and derive from being given this award that machine learning techniques and our long-term goal of increasing safety and productivity in ATM, as well as significantly reducing fuel consumption and CO2-emissions, well matches the expectations of the designated committee members. It certainly strengthens our confidence in MALORCA supports the focus of our project objectives, and serves as encouragement that the achieved progress towards the utilization of the proposed benefits is considered valuable for aviation.
Another remarkable work in the field of speech recognition technology was presented by Hunter Kopald from the MITRE Corporation. Together with his colleagues he elaborated on a research paper titled “Read Back Error Detection using Automatic Speech Recognition”. Without a doubt read-back and hear-back errors are a significant threat in air traffic control and lead to a relatively high number of incidents. Any monitoring system that serves to warn Air Traffic Controllers if a mismatch between a given instruction and the following read-back, or a missing correction of an incorrect read-back, has occured would be very beneficial for safety. However, in the presentation significant challenges were named relating to issues connected to the recognition of pilot transmissions and the requirement for highly sophisticated alert generation rules. Nevertheless, the already achieved results of recognition accuracy of controller transmissions of approximately 95% on average, and of pilot transmissions of approximately 85% on average, justify the continued pursuit of the desired project goals. MITRE plans to further improve the speech recognition component by adding real-time context information and will continue to investigate the types of read-backs that are acceptable to controllers. This task requires subject matter expertise from operational ATC staff.
The second topic (not only) MALORCA participants were curious about concerned machine-learning. With the general intention in mind of making air traffic more predictable for the benefit of all stakeholders, machine-learning models are provided to predict the future based on big data samples of past traffic flows assuming a level of determination that for some figures is not yet achieved. However, a common understanding existed amongst all participants in the seminar that figures like “requested time above fix/threshold”, “target/actual take-off time”, and many others need to be predicted and met more reliably/precisely in the near future. In those frequent cases where the traffic flow for whatever reason does not evolve as predicted those deviations need to be recognized instantly leading to a permanent recalculation of the whole model, to give an opportunity for managing all changes where required.
For increased productivity on the side of Air Navigation Service Providers special algorithms were provided to better sectorize airspace for the sake of improved traffic load balancing, taking into account not only occupancy values but also traffic complexity, and by doing so balancing the workload of Air Traffic Controllers. Last but not least machine-learning techniques were also provided to automatically analyze large collections of text data such as the Aviation Safety Reporting System contains. One can easily imagine that this is extremely helpful in an attempt to draw the right conclusions from thousands of occurrence reports on record for the benefit of safety.
Papers to be named in the above context are “A Comparative Analysis of Models for Predicting Delays in Air Traffic Networks” by experts from the Massachusetts Institute of Technology (MIT), the also award winning work “A Novel Machine Learning Model to Predict Abnormal Runway Occupancy Times and Observe Related Precursors” by the airport research unit of Eurocontrol, “Learning Air Traffic Controller Workload from Past Sector Operations” by Air Transport Economics and Management (ENAC) and the University of Toulouse, France, as well as “Topics and Trends in Incident Reporting – Using Structural Topic Modeling to Explore Aviation Safety Reporting System Data” by the RAND Corporation, Santa Monica, USA.
A multitude of other interesting papers and presentations can be found here.
Beyond all presentations and speeches that were given during the days of the seminar it was a great opportunity for the whole MALORCA-team on site to hold talks to many experts from all parts of the world and discuss the status quo, further steps, as well as future opportunities and potential benefits of Assistant Based Speech Recognition. Having the opportunity to look at the topic from the often very different perspectives of all stakeholders in the value chain of the aviation industry was incredibly informative. Many Air Navigation Service Providers, including some COOPANS-members, were represented by experts with extensive knowledge and experience who furthermore are substantially empowered by their companies to make strategic decisions. Therefore, in the context of dissemination, the seminar was very valuable for the MALORCA project and for the specification of the appropriate way ahead towards our challenging goals.